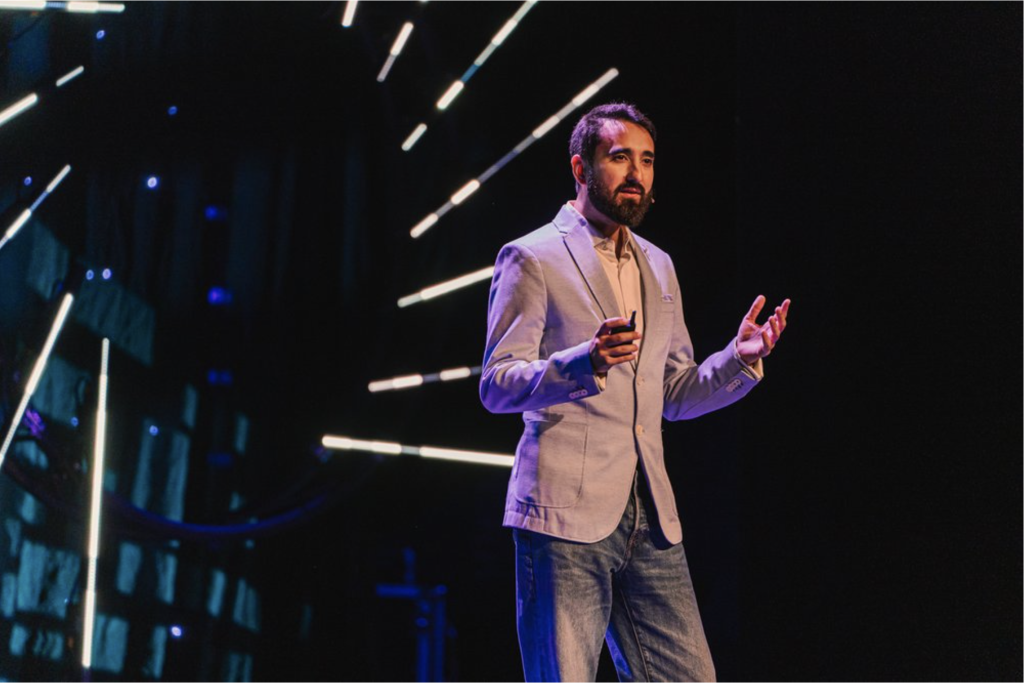
FAQs
Why is AI important in clinical discovery?
AI is important in clinical discovery because it can help identify unique and unusual cases that may lead to new medical breakthroughs. By analyzing large datasets more quickly and efficiently than humans can, AI can augment the ability of clinicians to find potential clinical discoveries that might otherwise be missed.
How does AI differ from traditional methods of clinical discovery?
Traditional methods of clinical discovery often rely on chance observations made by clinicians. AI, on the other hand, systematically analyzes large amounts of data to identify outliers or unexpected results that could lead to new insights. This moves the process from being accidental to intentional and systematic.
What are some historical examples of accidental discoveries in medicine?
Examples include the discovery of Aspirin’s heart attack prevention properties, Propranolol’s use as a beta-blocker for hypertension, Botox’s cosmetic application, Sildenafil’s (Viagra) effects, and Semaglutide’s (Ozempic) weight loss properties. These discoveries were all made by chance during investigations for other uses.
How can AI help prevent the missed opportunities for medical discoveries?
AI can screen thousands of patient records to identify outliers or unusual cases that might suggest a novel clinical discovery. This reduces the reliance on chance and increases the likelihood of uncovering important medical insights more systematically.
What are some potential risks of using AI in clinical research?
Potential risks include the possibility of AI systems amplifying existing biases, as seen in cases like the COMPAS algorithm in criminal justice. There are also concerns about AI’s accuracy in critical decisions, such as predicting sepsis or determining when rescue therapy is needed, where mistakes could have serious consequences.
What principles should guide the implementation of AI in clinical research?
The key principles include transparency, simplicity, and augmentation. AI systems should be transparent in how they work, simple to use, and designed to augment human abilities rather than replace human roles in clinical research.
How does the FDA view the use of AI in drug development?
The FDA has identified several areas where AI is being applied in drug development, including recruitment, site selection, data management, adherence tracking, data analytics, endpoint assessment, and post-market safety surveillance. The FDA is also working on developing standards and guidelines for the use of AI in these areas.
What is the ultimate goal of using AI in healthcare and clinical research?
The ultimate goal of using AI in healthcare and clinical research is to augment human capabilities, allowing healthcare professionals to focus more on patient care and scientific inquiry while AI handles tasks like data analysis and management. This aims to make clinical discovery and development more efficient, personalized, and patient-centered.